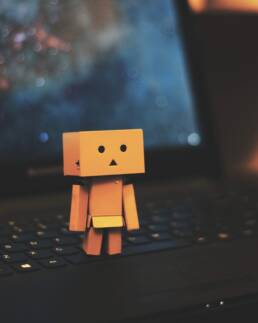
AI, FROM SENTIMENT ANALYSIS TO PREVENTION OF DEPRESSION
Online and distance learning can reduce emotional feedback from learners and the perception of the class sentiment.
At the same time, teachers can have a lot of digital data, especially text, coming from students’ assignments, personal essays, diary entries and others. AI can support the analysis of this large amount of data and in case of need can help teachers to monitor and predict the class sentiment.
Sentiment analysis attempts to automatically identify and recognize opinions and emotions in text, identifying if it represents a positive or negative opinion. These kinds of AI tools recognise the most common expressions of emotion such as happiness, sadness, anger, etc. You can understand how sentiment analysis works by reading this paper.
You can try how these systems work using a simple demo for emotion analysis of texts such as Depechemood. You can type, copy/paste your text and get the related emotions.
These AI based technologies can be used, on the contrary, also for awareness raising processes on the others’ perception of some kind of sentences or texts. This can encourage, for example, good online behaviours, consciousness of others’ perception and even prevention of cyberbullying.
An exercise you can do with your students is to observe how perceived emotions change if you replace some words. This can demonstrate how some words can generate good or bad emotions.
A more sophisticated AI for text analysis, machine learning, was used with excellent results in detecting the presence of depression in people. Depression is noticeable in the way you speak and express yourself in writing. The main assumption, confirmed by several researchers, is that depression leads to clear and consistent differences in language between those with and without symptoms of depression.
A research, published in Clinical Psychological Science, identified specific classes of words that can help predict whether someone is suffering from depression. These results have been reached through the analysis of a huge amount of data, made possible by AI that can actually process extremely large data banks in minutes. This can help spot linguistic features which humans may miss, calculating the percentage prevalence of words and classes of words, lexical diversity, average sentence length, grammatical patterns, and many other metrics.
Other models such as this one published by MIT can detect words and intonations in conversations that may indicate depression. Although these methods are accurate, there’s a limit to them as they depend on specific answers to specific questions to make the diagnosis.
It would not be surprising to see the implementation of such a system in our schools in the near future.